Concordance analysis is still something that humans do best
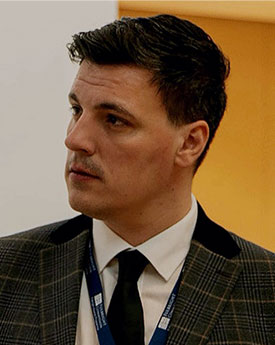
Concordance analysis is still something that humans do best
Author: Gavin Brookes
Published: 28 November 2024
Concordancing is, in my view, the most important technique in the corpus linguist’s toolkit. Essentially, it offers us a different way of looking at the language in a corpus. This ‘way of looking’ can offer valuable insight into the texts we are studying, helping us to identify features and patterns that we might not have expected to encounter. This is because the ‘vertical’ view of language use that concordancing affords allows us to see the language used in our corpus in a different way, and this can help us to spot features of that language use that we might have missed had we only read that language use in a more linear, ‘horizontal’ way.
So, concordancing can enrich a corpus analysis and help us to arrive at potentially more interesting or exciting insights about language use. But I (and I’m sure, many others!) think that the importance of concordancing to corpus linguistics is actually more fundamental than this. That’s because concordancing brings us closer than any other corpus linguistic technique to the textual reality of the language use captured in our corpus. It is these textual realities that sit behind any numerical patterns or statistical relationships on which we might base our analytical claims. So, the opportunity to engage with such textual realities is essential, in my view, for testing our hypotheses about the quantitative patterns we observe in the data, and ultimately for accounting for those patterns and explaining why they are interesting or worth talking about.
For most people working in corpus linguistics, this kind of engagement with textual reality, enabled in the main by concordancing, has been a cornerstone of their methodologies. And in taking a critical look at other digital methods that might be viewed as supplementing – or even replacing – corpus linguistic techniques (e.g., topic modelling [1], LIWC [2], culturomics [3], inter alia), it seems that this concern for looking closely at textual context is not always shared by users of these other methods. When this engagement with textual reality takes place, this can, as noted, helpfully give us another view of the language in our corpus. On the other hand, the lack of such engagement can unhelpfully steer us away from textual reality, with observations being based just on automatically generated abstractions of the data, with the risk that such abstractions might (and can) bear little correspondence to textual reality. Arguably, we might even be able to say that, broadly put, looking or not looking at textual context can be the difference between an analysis that introduces these other approaches into a corpus analysis in a way that is more or less helpful.
The picture that I’ve painted so far makes it clear (I hope!) just how beneficial – essential, even – concordancing is to the analysis of a corpus. However, in reality, analysing concordance lines can be challenging. Of course, the more frequent the word or feature that you want to analyse is, the more demanding the task of analysing concordance lines becomes, in terms of both time and energy. The scale of the task then grows even more if we want to look at multiple items or expand the concordance beyond the simple concordance line (both of which are likely), or if we encounter so-called ‘false starts’ or ‘dead ends’ in our analysis, but only reach that point having looked at hundreds of concordance lines (which also happens…).
For these reasons, it is understandable that some might be daunted by the prospect of concordance analysis, and therefore look for ways of avoiding or at least automating it. For a recent paper I published with Niall Curry (Manchester Metropolitan University) and Paul Baker (Lancaster University), we were interested in looking at how the popular generative AI tool, ChatGPT, might be able to support corpus analysis in this sort of way [4]. We set out to replicate parts of three separate corpus-based discourse projects. We compared the tool’s performance against human-led approaches, and we wanted to evaluate how well ChatGPT could analyse keywords, concordance lines, and extended text samples larger than concordance lines. Like with the publications cited above, we took a critical approach to our evaluation, but we were open-minded about what the tool might be able to do, and about how it might be able to help.
The first task involved semantically categorising 72 keywords from online support group discussions around mental health, based on an analysis by Hunt and Brookes [2]. Without sight of the data, ChatGPT was able to generate ten categories, slightly fewer than the eleven created by human analysts, with some overlap. For instance, both analyses included similar groupings like ‘Food and eating’ / ‘Dietary factors’ and ‘Feelings and emotional responses’ / ‘Emotional and psychological aspects’. However, ChatGPT tended to categorise words based on their surface meanings rather than their nuanced, context-specific uses. For example, terms like ‘problem’ and ‘problems’ were categorised by ChatGPT under ‘Emotional and psychological aspects’, while human analysts grouped them under ‘Diabulimia and disordered behaviours’, due to their euphemistic application in context. Overall, the AI-generated categories were often generic, lacking the granularity necessary for analysing specialised discourse. Although further iterations led to slightly improved categorisation, meaningful contextualisation still required human intervention.
The second task replicated part of a study by Baker et al. [5], focusing on how UK national newspapers represented the relationship between Islam and homosexuality. Human analysts manually examined 106 concordance lines of the word ‘homosexuals’, identifying eight instances where homosexuality was linked to Islam, with two categories emerging from the analysis: (1) Islam depicted as homophobic and (2) Islam and homosexuality both represented as similarly oppressed groups. ChatGPT struggled much more with this task than with the keyword categorisation. For example, it identified seven instances where it purportedly found links between Islam and homosexuality, but these instances differed completely from the ones highlighted by human analysts. To illustrate, one of the lines identified by ChatGPT was ‘Cote du Rhone and wondered why it should be the case that homosexuals have unusually long index fingers’, a line that has no evident relevance to Islam and homosexuality, illustrating the tool’s failure to accurately pinpoint meaningful connections. There was also evidence of misinterpretation and erroneous grouping. For instance, ChatGPT grouped the seven instances it identified into five categories, despite having only a few examples per category, suggesting limited analytical coherence. It described one category as ‘Hate and Discrimination’, linking it to a single concordance line, which claimed to reflect stereotypes or perceptions of Islam, even though the line did not explicitly mention Islam. This demonstrates an overreach and potential misinterpretation of the context. In another example, ChatGPT cited the line ‘made in favour of repealing the law that it encourages hatred of homosexuals’ as related to Islam when the context was unrelated to Islamic discourse but rather it referred to British legislation. You can see all of the concordance lines that ChatGPT identified as linking homosexuality to Islam, below.
Cote du Rhone and wondered why it should be the case that | homosexuals | have unusually long index fingers; three pregnant wives and one post- |
Shah and Islam, it was made clear that | homosexuals | do form a social group for the purpose of claims for asylum |
last year, offers legal recognition for all cohabiting couples, including | homosexuals | Hailed by supporters as France’s first truly modern piece of” |
made in favour of repealing the law that it encourages hatred of | homosexuals | Hindus and Sikhs.” In 1994 the age of consent for homosexuals was lowered from 21 to 18. Downing Street said equality was |
(www.morocco-travel.com). Legislation to reduce the age of consent for | homosexuals | in England, Wales and Scotland to 16 reached the statute book last night after |
stupendously incorrect politically, referring to women as ‘bitches’ and | homosexuals | as ‘benders’. A disproportionate percentage of his streetwise patois |
Aside from its analytical utility, there were also more fundamental issues concerning data integrity and fabrication that emerged from how ChatGPT examined the concordance lines. ChatGPT modified the input data (i.e., the concordance lines) during the analysis, for example by altering the wording in concordance lines when providing responses, such as replacing the word ‘made’ with ‘move’. This is, of course, antithetical to maintaining data integrity – a critical tenet of empirical research. Also concerning data integrity, ChatGPT seemed to conflate separate concordance lines, drawing from different parts of the text to construct interpretations that did not, in (textual) reality, reflect the original data. Another limitation with implications for empiricism concerned the non-deterministic nature of the analysis, with further attempts at reanalysis showing inconsistency. In particular, when prompted to review the same concordance lines again, ChatGPT produced a completely different set of results. This raises significant concerns about not only the reliability but also the repeatability of the analyses it can give us.
Aside from its analytical utility, there were also more fundamental issues concerning data integrity and fabrication that emerged from how ChatGPT examined the concordance lines. ChatGPT modified the input data (i.e., the concordance lines) during the analysis, for example by altering the wording in concordance lines when providing responses, such as replacing the word ‘made’ with ‘move’. This is, of course, antithetical to maintaining data integrity – a critical tenet of empirical research. Also concerning data integrity, ChatGPT seemed to conflate separate concordance lines, drawing from different parts of the text to construct interpretations that did not, in (textual) reality, reflect the original data. Another limitation with implications for empiricism concerned the non-deterministic nature of the analysis, with further attempts at reanalysis showing inconsistency. In particular, when prompted to review the same concordance lines again, ChatGPT produced a completely different set of results. This raises significant concerns about not only the reliability but also the repeatability of the analyses it can give us.
So, we found that ChatGPT’s performance was somewhat inconsistent across the tasks. It showed some potential for grouping keywords at a surface level but failed to provide meaningful, context-sensitive analyses for more complex tasks such as examining concordance lines and carrying out functional categorisation. In other words, as we provided more textual context to the tool for its analysis (i.e., moving beyond a list of keywords and towards concordance lines and text extracts), the weaker it performed. It seemed to us that, as the analysis became more context-dependent, the more apparent it became just how superficial and surface-level ChatGPT’s analysis was. In addition to this, cutting across all of the analyses we asked it to do, we were left with pressing concerns about data modification, inconsistent analytical processes, and a lack of transparency in the analysis arising from the tool’s non-deterministic nature. Of course, generative AI is developing apace, and these kinds of results will need to be tested in time, to understand whether and how tools such as this have become more sophisticated in ways that are useful for empirical linguistic research. For now, though, challenges related to data ethics, transparency and replicability remain significant hurdles.
If it is to be used to support concordance analysis, ChatGPT is perhaps best suited as a supplementary tool, to be used only under (very close) human supervision. I’d like to conclude this blog with a couple of questions that occur to me at this point. The first is, if we have to check through all of the results that they produce, then are generative AI tools really saving us much time, if any at all? I doubt that it does. The second is, is concordance analysis too important to be left up to the limitations and fallibilities of generative AI tools? I think that it is. So for me, for now at least, concordance analysis is still something that humans do best.
References
- Brookes, G., & McEnery, T. (2019). The utility of topic modelling for discourse studies: A critical evaluation. Discourse Studies, 21(1), 3-21. https://doi.org/10.1177/1461445618814032.
- Hunt, D., & Brookes, G. (2020). Corpus, Discourse and Mental Health. Bloomsbury. https://www.bloomsbury.com/uk/corpus-discourse-and-mental-health-9781350059184/.
- Brookes, G., & McEnery, T. (2020). Corpus linguistics. In S. Adolphs & D. Knight (Eds.), The Routledge handbook of English language and digital humanities (pp. 378–404). Routledge. https://www.taylorfrancis.com/chapters/edit/10.4324/9781003031758-20/corpus-linguistics-gavin-brookes-tony-mcenery.
- Curry, N., Baker, P., & Brookes, G. (2024). Generative AI for corpus approaches to discourse studies: A critical evaluation of ChatGPT. Applied Corpus Linguistics, 4(1), 100082. https://doi.org/10.1016/j.acorp.2023.100082.
- Baker, P., Gabrielatos, C., & McEnery, T. (2013). Discourse Analysis and Media Attitudes: The Representation of Islam in the British Press. Cambridge University Press. https://doi.org/10.1017/CBO9780511920103.
- Curry, N. (2021). Academic Writing and Reader Engagement: Contrasting Questions in English, French and Spanish Corpora. Routledge. https://www.routledge.com/Academic-Writing-and-Reader-Engagement-Contrasting-Questions-in-English-French-and-Spanish-Corpora/Curry/p/book/9781032011134.