Laurence Anthony: Breaking New Ground: AI-Enhanced Concordance Analysis
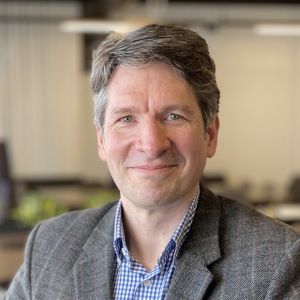
Note: This post is a mirror of the original article first published on the University of Birmingham webpage.
Laurence Anthony: Breaking New Ground with AI-Enhanced Concordance Analysis
Author: Alexander Piperski
Published: Posted on 28 October 2024
In this guest post, Laurence Anthony, Professor at Waseda University (Japan) and a member of the RC21 advisory board, summarizes his talk on concordance reading at the ICAME 45 conference and discusses the future of this technique.
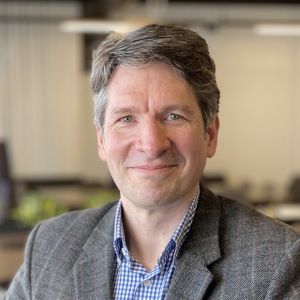
In the RC21 workshop at the ICAME 45 conference held in Vigo, Spain, I had the honor and pleasure to present my latest research on an innovative approach that could transform how we conduct concordance searches. By integrating artificial intelligence—specifically, transformer-based word and sentence embeddings—with traditional concordance methods, I’ve been working to address long-standing limitations in corpus analysis while exploring exciting new possibilities for both researchers, teachers, and language learners.
From Challenge to AI Solution
For many years, I’ve observed two significant limitations in traditional concordancing. First, corpus researchers often need to craft intricate search queries to capture various language usage patterns, accounting for spelling variations, synonyms, and idiomatic expressions. It can make the queries increasingly complex. Second, most of the corpus software tools available to researchers, teachers, and learners sort results alphabetically, requiring users to manually scan through concordance lines to identify meaningful patterns. While some of my previous innovations like ‘KWIC patterns’ (Anthony, 2018; 2020) have improved this scanning process, analyzing large datasets remains challenging.
This is where AI technology can help. In the talk, I explained how I use transformer-based word and sentence embeddings in a “fuzzy” querying approach to allow users to perform synonym, semantic, and register variation searches, without constructing complex search strings. The approach also allows for interesting groupings and rankings of concordance results to reveal patterns that might otherwise have remained hidden.
In case studies using the BE06 and AmE06 corpora, I demonstrated three key applications:
- Synonym searches: Finding how words like “car” and its synonyms are used in natural language without listing every possible variant.
- Semantic groupings: Identifying uses of the word “bank” and separating cases related to money from those related to rivers.
- Language variety matching: Identifying equivalent expressions between British and American English, such as finding the US equivalent of “This struck me as a strange state of affairs.”
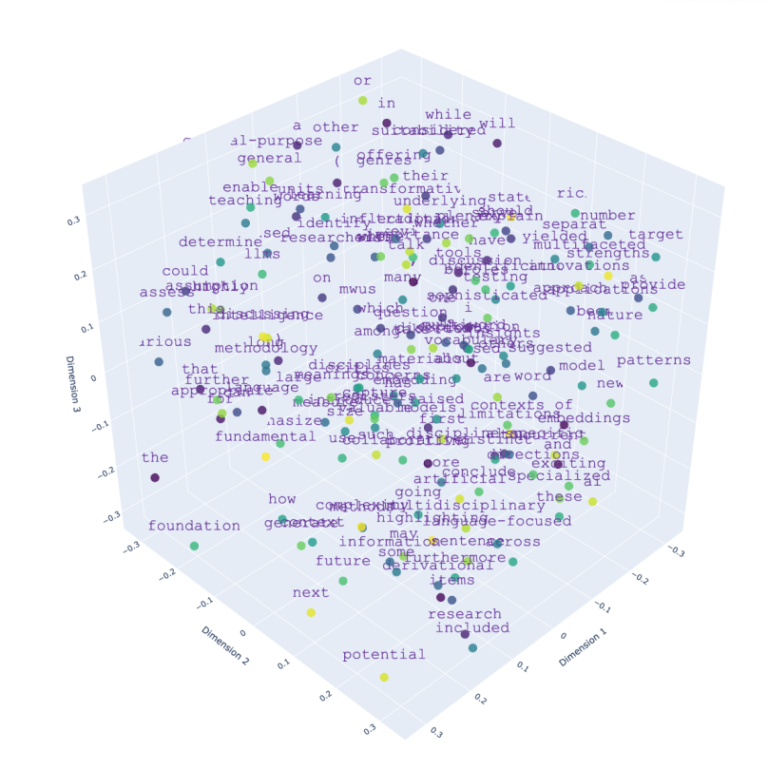
A New Frontier in Corpus Linguistics
Every day, we’re seeing rapid improvements in AI technology. Rather than feeling intimidated, I’m excited about its potential to revolutionize how we understand language. What new insights into language might AI tools help us uncover? How might AI evolve to support even more sophisticated analyses? Can we use AI to develop more accessible tools for language teachers and learners? These are the questions that drive my research forward.
In working on this project, I’ve come to see Large Language Models (LLMs) and other AI developments as more than just technological advancements—they provide us with a completely new lens through which we understand and analyze language. For those of us passionate about linguistics, whether we’re researchers, educators, learners, or just enthusiasts, this is a truly remarkable new era.
References
- Anthony, L. (2018). Visualization in corpus-based discourse studies. In C. Taylor & A. Marchi (Eds.), Corpus approaches to discourse: A critical review (pp. 197–224). Routledge.
- Anthony, L. (2022). What can corpus software do? In A. O’Keeffe & M. McCarthy (Eds.), The Routledge handbook of corpus linguistics (pp. 237–276). Routledge.